Advanced RAG for Enhanced and Optimized Results
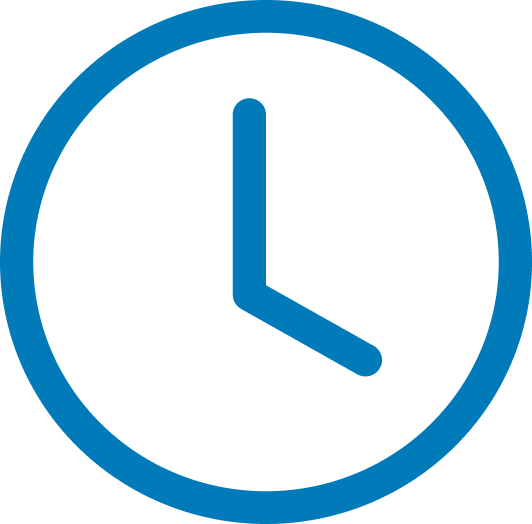
June 19, 2024
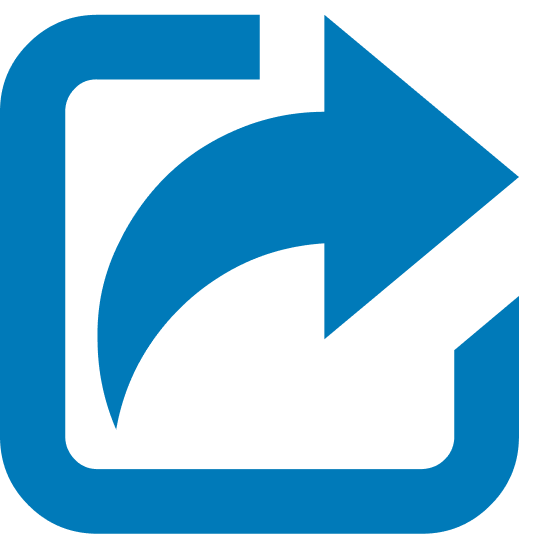
Overview
LLMs can be very inconsistent and unpredictable. They often get the answers correct but are also susceptible to hallucinations. The answers which they form make no sense.
RAG (Retrieval Augmented Generation) is an AI Framework that has been on the rise for answering the questions present in external data not trained on by the LLM. RAG models have been very popular in the past year for these tasks. These models can leverage large external knowledge sources like websites, databases or document collections to improve their ability to generate accurate and informative answers.
An RAG model is made up of two parts: a retriever and a generator.
- The retriever is responsible for recognizing and retrieving all the relevant documents from the external data source.
- The generator uses these documents as context, along with the input query to generate the response.
Today we take a deeper dive into every individual step involved in an RAG model.
Advanced RAG
High Level Diagram
Dataset
For this research, we have utilized a proprietary dataset consisting of company policies, processes, methodologies, and project information, including client data and tech stacks. This comprehensive dataset was sourced from our company's website and cleaned using the Beautiful Soup library for web scraping and data parsing.
The decision to use an internal dataset was driven by the need to leverage domain-specific knowledge and terminology relevant to our organization's operations. By training our models on this curated dataset, we aimed to enhance their understanding and generation capabilities within the context of our company's practices and workflows.
Chunking
Chunking is the process of breaking down large documents into smaller chunks.
RAG models operate on individual messages instead of entire documents that make them computationally efficient and allow for targeted retrieval.
By breaking down documents into smaller chunks, RAG models can quickly retrieve relevant passages without having to process the entire document, thereby improving performance and scalability.
There are many types of chunking methods like fixed size chunking, semantic chunking, document based chunking, etc. Each method is specific to one’s own use case. For our purpose we have used Recursive chunking.
Recursive Chunking: This method considers the structure of the documents while segregating it into chunks. In recursive chunking, we divide the text into smaller chunks in a hierarchical and iterative manner using a set of separators. If the initial attempt at splitting does not produce chunks of the required size, the method recursively calls itself on the resulting chunks with a different separator until the desired chunk size is achieved.
For eg. langchain framework offers RecursiveCharacterTextSplitter class, which splits text using default separators (“\n\n”, “\n”, “ “,””)
Embeddings and Embedding Models
Essentially, they're mathematical representations of words in a high-dimensional space. Embeddings refer to dense, continuous vectors representing text in a high-dimensional space. These vectors serve as coordinates in a semantic space, capturing the relationships and meanings between words. In the context of LLMs, embeddings play an important role in retrieving the right context for RAG.
Embedding models are ML models that are trained to generate these dense vector representations. There are various kinds of embedding models, each designed to capture embeddings at different levels of granularity. (For eg. word-level, sentence-level, document-level).
There are various different types of embedding models available these days which cater to different requirements.
For our use case, we have chosen OpenAI’s ‘text-embedding-3-small’ embedding model, as it is great for document retrieval and has a low cost. It produces an embedding of size 1536. The model’s score on a commonly used benchmark for multi-language retrieval (MIRACL) is 44% and on MTEB(Massive Text Embedding Benchmark) (a benchmark for English tasks) is 62.3%.
Indexing and Vector Stores
Vector indexes allow users to efficiently query and retrieve vector embeddings from large datasets. The choice of vector store depends on the specific use case and requirements.
Below are the different indexing options that we have for indexing
HNSW (Hierarchical Navigable Small World): A graph based indexing, use this for higher speed and decent accuracy. eg. for image search and document retrieval systems
DiskANN: Used when the dataset is enormous and essentially cannot be placed within the RAM. eg. large-scale image.
IVF (Inverted File Index): Effective for very large datasets where reducing computational cost is necessary without significantly compromising search accuracy. Divides the search space into quantized regions or clusters, significantly reducing the search area by focusing on the most promising regions.
Flat indexing: Flat indexing, often referred to as "brute force" search, involves directly comparing a query vector against every vector in the dataset to find the nearest neighbors without any form of pre-processing or partitioning of the data.
We have analyzed below 4 vector stores and the indexing options that they provide.
Document Retrieval
Retrieval of documents is an integral part of an RAG model. This retrieval is done by functions called retrievers. These are responsible for retrieving relevant documents from a knowledge base and then rank them based on similarity. This information can then be used by the LLM to generate the final output. Before we jump into retrievers, let us explain the term Reranking.
Reranking in RAG
Sometimes, the initial retrieval step can be a little noisy, which results in low-quality or irrelevant documents being passed to the model. This can lead to performance that is suboptimal. Reranking is a technique employed in RAG models which improves the quality of documents retrieved before they are processed by the language model.
The Reranking Process
- Initial Retrieval: The retriever performs a first-pass retrieval to obtain a set of candidate documents relevant to the input question.
- Reranking: A reranking model takes the question and the candidate documents as input. It scores each document based on its relevance to the question. The documents are then resorted based on these reranking scores.
- Top-k Reranked Docs: The top-k reranked documents are selected and passed to the language model for answer generation.
The reranking model is typically a cross-encoder that attends to the full question-document pair to produce a relevance score. This allows the model to capture deeper semantic relations between the question and each document.
Reranking improves answer quality by prioritizing relevant documents and mitigating noise, and retrieval errors. It allows language models to focus on high-quality sources. Although adding computation, reranking generally enhances open-domain question-answering performance over using only initial retrieved documents.
Query: What is CB Training policy?
Without reranker:
The CB Training policy covers certification courses that enhance productivity at CoffeeBeans. It includes in-house training, periodicals, leadership programs, and enterprise licenses provided by CoffeeBeans. There are two main categories: CB suggested training and individual choice certification. The budget for training will vary each financial year. For more details, you can refer to the Training & Development Policy document available at https://wiki.coffeebeans.io/PeopleDocs/T&Dpolicy.
With reranker:
Nano:
The CB Training policy at CoffeeBeans covers certification courses that enhance productivity for both technical and non-technical roles. There are two main categories under the training program: CB suggested training and individual choice certification. CB suggested training includes in-house training, periodicals, leadership programs, and enterprise licenses provided by CoffeeBeans. Individual choice certification allows employees to choose their own certification courses within the allocated budget.
Small:
The CB Training policy at CoffeeBeans covers certification courses that enhance productivity for both technical and non-technical roles. There are two main categories under the training program: 1. CB suggested training which includes in-house training, periodicals, leadership programs, and enterprise licenses provided by CoffeeBeans. 2. Individual choice certification for which employees can select courses based on their preferences.
Medium:
The CB Training policy at CoffeeBeans covers certification courses that enhance productivity for both technical and non-technical roles. The training program includes in-house training, periodicals, leadership programs, and enterprise licenses provided by CoffeeBeans. There are two main categories: CB suggested training and individual choice certification.
Large:
The CB Training policy at CoffeeBeans covers certification courses that enhance productivity for both technical and non-technical roles. It includes in-house training, periodicals, leadership programs, and enterprise licenses provided by CoffeeBeans. There are two main categories: CB suggested training and individual choice certification.
Query: What is at the highest priority for CB right now? Answer in 100 words
Without reranker:
Based on the provided information, at the highest priority for CB right now is likely a focus on enhancing the organization's branding and growth through initiatives such as representing CB in various tech/business forums, becoming a Tech Evangelist, and contributing to white papers and open source projects. Additionally, driving tech discussions in Pre-Sales and ensuring strong competence in hiring, mentoring, and training are key priorities. Furthermore, for the DEV team, delivering on engineering and agile practices, while for the BA team, stakeholder management and functional expertise are crucial. Overall, a balance of technical innovation, leadership development, and organizational growth appears to be the primary focus for CB.
With reranker:
Nano:
CB is focusing on initiatives such as representing the organization in various tech and business forums, being a Tech Evangelist, writing and publishing white papers, contributing to open source projects, and driving tech discussions in Pre-Sales. Additionally, strong competence in hiring, mentoring, and training is emphasized to ensure the team's growth and success. In terms of engineering and agile practices, the focus is on delivering end-to-end projects, mastering all practices, tweaking and restructuring when needed, making difficult decisions when required, and ensuring timely completion within budgets.
Small:
CoffeeBeans is currently focusing on initiatives such as representing the organization in various tech and business forums, becoming a Tech Evangelist, writing and publishing white papers, contributing to open source projects, and driving tech discussions in Pre-Sales to enhance branding and growth.
Key priorities within the organization include strong competence in hiring, mentoring, and training, mastering engineering practices to deliver projects efficiently, having leadership qualities to make tough decisions when needed, ensuring end-to-end delivery of multiple projects, managing stakeholders effectively, aligning engineering efforts with business objectives, and actively seeking feedback for continuous improvement in engineering outcomes.
Medium:
Currently, the highest priority for CoffeeBeans in terms of organizational focus seems to be enhancing branding and growth through various initiatives. Some of the key approaches include representing CoffeeBeans in various tech/business forums, being a Tech Evangelist for CoffeeBeans , writing/publishing white papers, contributing to open source projects, and driving tech discussions in Pre-Sales. By engaging in these activities, CoffeeBeans aims to increase visibility, credibility, and thought leadership in the industry, ultimately leading to enhanced branding and growth opportunities.
Large:
CoffeeBeans's current highest priority in terms of organizational focus is on enhancing brand visibility and growth. Key initiatives being pursued include representing CoffeeBeans in various tech/business forums, acting as a Tech Evangelist for CoffeeBeans by writing and publishing white papers, contributing to open source projects, and driving tech discussions in Pre-Sales. Additionally, efforts are directed towards introducing initiatives that promote branding and growth, such as participating in outreach pitches, stakeholder management, and becoming subject matter experts for clients. These initiatives aim to strengthen CoffeeBeans's presence in the market and drive growth through effective branding strategies.
Retrievers for Information Retrieval
Introduction
Retrieval systems play a crucial role in information retrieval tasks, allowing users to find relevant documents from large collections. In this whitepaper, we explore two primary types of retrievers: Dense Retriever and Sparse Retriever. Each type has distinct characteristics and is suited for specific use cases.
1. Dense Retriever
Dense retrievers focus on capturing semantic relationships between queries and documents. Here are the key features of Dense Retrievers:
1.1 Similarity Search
Dense retrievers perform a similarity search between the query vector and document vectors.
- They use dense representations (such as embeddings) to measure similarity.
- The goal is to retrieve the most relevant documents based on semantic content.
1.2 Use Cases
Open-domain question answering: Dense retrievers excel at finding relevant passages or documents for answering user queries.
Semantic search: They enhance search results by considering context and meaning.
1.3 Example: FAISS Retriever
- FAISS (Facebook AI Similarity Search) is a popular library for efficient similarity search.
- It leverages dense vector representations and indexing techniques to achieve fast retrieval.
2. Sparse Retriever
Sparse retrievers focus on exact keyword matching between queries and documents. Here are the key features of Sparse Retrievers:
2.1 Keyword Matching
Sparse retrievers compare query terms directly with document terms.
They use inverted indexes to quickly identify relevant documents.
Exact matching is crucial for these retrievers.
2.2 Use Cases
Legal and medical domains: Sparse retrievers are effective when precise keyword matching is essential.
Specific information retrieval tasks: They work well for finding specific documents based on keywords.
2.3 Example: BM25 Retriever
BM25 (Best Matching 25) is a popular probabilistic retrieval model.
It considers term frequency, document length, and inverse document frequency for ranking documents.
Ensemble Retriever
Ensemble retrievers address this by combining the strengths of distinct retrieval methods, thereby improving overall retrieval performance. This retriever takes a list of retrievers as input, ensembles the results and reranks them based on the Reciprocal Rank fusion algorithm. We won’t discuss the complete working of the reciprocal rank fusion algorithm, but in short, this algorithm is used to combine the results of multiple retrieval systems. It reranks them in a way where documents ranked higher by different retrievers are given more weightage.
A popular ensemble approach is to combine the FAISS and BM25 retrievers. FAISS excels at capturing semantic relevance, while BM25 is adept at finding exact keyword matches. By merging their results, the ensemble retriever can leverage the strengths of both techniques – retrieving semantically relevant documents as well as those with precise keyword matches. This combination enhances the overall recall and precision of the retrieval system.
For eg.
Query: Tell me about the tech stack of Amaka
Answer (with only FAISS Retriever)
The tech stack for AMAKA Studio includes the following technologies:Frontend:- React- TypeScriptMaterial-UI Backend- Strapi- PostgreSQL- Node.js- JavaScript\Third-party libraries used in both frontend and backend- Flutterwave for payment gateway integration- Algolia for search engine functionalities, filtering, recommendations, and personalization'
Answer (with Ensemble Retriever, both FAISS and BM25)
The tech stack for Amaka includes the following technologies: Front-End: - React v18 - Material-UI - Editor.js - Editor Js-blocks-react-renderer - Flutterwave (for payment gateway integration) - Algolia (for search engine and functionalities like filters, recommendations, and personalization) Back-End: - Strapi - PostgreSQL - Node.js - JavaScript Third-Party Libraries: - Flutterwave (for payment gateway integration) - Algolia (for search engine and functionalities like filters, recommendations, and personalization) Infrastructure and Deployment Tools: - Docker - Jenkins These are the key technologies and tools used in the tech stack for Amaka.
Q2: Tell me about the training policy
Answer (with only FAISS Retriever)
The CoffeeBeans training policy covers in-house training for technical and non-technical roles, leadership programs, and enterprise licenses provided by CoffeeBeans . The enterprise license can include courses from platforms like Udemy, Microsoft, Coursera, etc. Individual choice certification is also supported, where individuals can opt for certified courses related to their skill set at work. Certified online webinars and workshops are covered under this program. Additionally, individuals can use the study material budget if the certification course budget exceeds, increasing coverage from 50% to 70%.
Answer (with Ensemble Retriever, both FAISS and BM25)
The CoffeeBeans Training Policy covers two main categories. CoffeeBeans Suggested Training: This includes in-house training (both technical and non-technical), leadership programs, and enterprise licenses provided by CoffeeBeans. The enterprise license can cover courses from platforms like Udemy, Microsoft, Coursera, etc. If you want to purchase an enterprise license, you need to get the budget details from the HR team before doing so.2. Individual Choice Certification: This allows individuals to opt for certified courses in their area of skill set at work. The course can be any certified course, including online webinars and workshops. If the budget for the individual certification course exceeds the allocated amount, the individual can use the study material budget to cover the additional cost.\n\nOverall, the training program aims to enhance productivity at CoffeeBeans for both technical and non-technical roles.
The answers produced by the Ensemble retriever are much more accurate and detailed.
Conclusion
Choosing the right retriever depends on your specific use case. Consider the trade-offs between semantic understanding (Dense Retriever) and precise keyword matching (Sparse Retriever) when designing your information retrieval system.
Multi-Query Retrieval Techniques
In information retrieval, a single query may not always suffice to capture the complexity of the required information or address all the intricacies of a task. Enter the concept of multi-query retrieval. This technique involves breaking down a complex query into multiple sub-queries, each focusing on a specific aspect of the overall query. These subqueries are then used to independently retrieve relevant documents from a knowledge corpus, and the retrieved results are combined to generate the final response.
1. The Multi-Query Approach
1.1 Breaking Down Complex Queries
- Multi-query retrieval recognizes that complex queries often involve multiple facets or subtopics.
- Instead of treating the entire query as a monolithic unit, it dissects it into smaller, more manageable sub-queries.
- Each subquery hones in on a particular aspect of the overall information needed.
1.2 Independent Retrieval
- Once the sub-queries are defined, they are used to retrieve relevant documents independently.
- The retrieval process can be based on various techniques, such as keyword matching, semantic similarity, or other relevance models.
- Each sub-query retrieves a subset of relevant documents.
1.3 Combining Results
- The retrieved documents from each sub-query are then combined to form the final response.
- This combination can involve merging the results, ranking them, or applying additional filters.
- The goal is to provide a comprehensive and accurate answer to the original complex query.
2. Advantages of Multi-Query Retrieval
2.1 Handling Ambiguity
- Queries often have multiple meanings or interpretations.
- Multi-query retrieval allows us to address each interpretation separately.
- By doing so, we improve the accuracy of retrieval from the knowledge base.
2.2 Improved Coverage
- Breaking down complex queries into simpler sub-queries ensures a more diverse set of retrieved documents.
- Each subquery focuses on a specific aspect, leading to better coverage of the required information.
- Users benefit from a broader range of relevant content.
2.3 Capturing Nuances
- Long and intricate queries may contain multiple sub-topics.
- Dividing the query into specific subqueries, where each subquery corresponds to a distinct subtopic, results in a more complete generated output.
- Nuances and fine-grained details are better captured.
For eg. Query: “What is the leave and sabbatical policy? Tell me about the architecture of XYZ”
Subqueries:
- “What is the leave policy?”
- “What is the sabbatical policy?”
- “Tell me about the architecture of XYZ?”
Each individual subquery is used to independently retrieve information from the corpus. The retrieved documents and then combined to form a comprehensive output covering all aspects of the base query.
The multi-query in most use cases can provide a more complete response, addressing all the topics involved in the base query.
Results: Multiquery proved to be helpful in cases with complex and long questions.
Q: What is the Posh policy? Who is aayushi and Mitesh? Tell me about the presales guide/process.
Without Multi-Query
The Prevention of Sexual Harassment (POSH) policy at CoffeeBeans is designed to provide a safe and harassment-free work environment. It defines sexual harassment at the workplace and outlines the company's redressal procedures. The policy applies to all individuals associated with CoffeeBeans.
Aayushi is the EA/Admin and Mitesh is the CTO of CoffeeBeans.
The presales guide/process involves creating relevant pitches, sharing knowledge through blogs and forums, orchestrating events like Hackathons, and identifying and fixing gaps in processes within the organization.
With Multi-Query
The Posh policy implemented by CoffeeBeans Consulting LLP is a company policy that defines sexual harassment at the workplace and outlines the procedures for addressing and resolving complaints. It applies to all individuals associated with CoffeeBeans, including employees, contractors, consultants, vendors, interns, and visitors. The policy establishes a Prevention of Sexual Harassment Committee (POSH committee) responsible for investigating complaints, ensuring a fair hearing, and making appropriate recommendations for action.
Mitesh is the CTO of CoffeeBeans.
Aayushi is a point of contact for the Staffing Tool and is responsible for queries related to it.
The Pre-Sales Guide is a comprehensive document that provides guidance on all Pre-Sales activities. It includes information on developing a Sales/Pre-Sales process, consulting with clients to understand their needs, evaluating existing technology infrastructure, recommending solutions, presenting technology solutions, managing vendor relationships, negotiating contracts, and staying up-to-date with emerging technology trends.
The guide also covers managing the technology budget, ensuring compliance with industry regulations and data protection standards, monitoring the technology landscape for opportunities, and continuously improving processes within the organization.
In addition, the Pre-Sales Guide mentions specific ways in which Pre-Sales professionals can contribute, such as creating relevant pitches, sharing knowledge through blogs and forums, orchestrating events like Hackathons, and identifying and fixing loopholes or gaps in processes.
The guide also provides an overview of the Presales and Inception Estimation Guide, which outlines the steps to conduct estimation during the Pre-Sales and Inception phases. This estimation helps in determining release and delivery dates, creating a product roadmap, and calculating costs and team size.
The guide also mentions a Standard Operating Procedure (SOP) for Pre-Sales activities, which includes identifying the skills and experience level required for estimations, assigning a team for the Pre-Sales activity, and having a backup team in case of emergencies. Estimations are to be delivered as per deadlines received from the Sales team.
Overall, the Pre-Sales Guide serves as a comprehensive resource for Pre-Sales professionals, providing them with the necessary information and guidance to effectively carry out their activities and contribute to the success of the organization.
Long-term and Short term memory
For memory, we use the ChatOpenAI API from langchain which is capable of engaging in context-aware conversations. The ChatOpenAI model can be used as the generation component, responsible for generating natural language responses based on the retrieved relevant information and the current conversation context.
We also use 3 different classes from langchain’s schema, which define data structures for representing conversational messages.
- SystemMessage represents system-level messages that provide instructions or set the context for the conversation.
- HumanMessage represents messages sent by the user.
- AIMessage represents messages generated by the AI system (in this case, the RAG system).
The retrieved information, along with the conversation history (represented by a list of SystemMessage, HumanMessage, and AIMessage objects), is provided as input to the generation component (ChatOpenAI).
Document Retrieval with Meta-Data Filtering
In information retrieval systems, the relevance of retrieved documents can be significantly improved by incorporating meta-data filtering. When documents are tagged with additional information (metadata), we can leverage this data to narrow down search results. In this whitepaper, we explore the concept of meta-data filtering and its benefits.
1. What is Meta-Data Filtering?
1.1 Definition
- Meta-data filtering is a technique used to enhance document retrieval.
- It involves leveraging additional information associated with documents (metadata) to filter out irrelevant results.
- By considering metadata, we can improve the precision and relevance of retrieved documents.
1.2 Examples of Metadata
- Dates and Times: Documents may have creation dates, modification dates, or publication timestamps.
- Genres and Categories: Metadata can include genre labels (e.g., “science fiction,” “historical,” “romance”) or specific categories (e.g., “research papers,” “news articles”).
- Names and Types: Metadata may contain author names, document types (e.g., PDF, HTML), or file formats.
- Descriptions and Keywords: Descriptive metadata provides additional context about the content.
- Custom Fields: Depending on the use-case, custom metadata fields can be defined (e.g., project IDs, client names).
2. Advantages of Meta-Data Filtering
2.1 Improved Relevance
- By filtering based on metadata, we exclude documents that do not match specific criteria.
- Users receive more relevant results tailored to their information needs.
2.2 Handling Ambiguity
- Ambiguous queries can benefit from metadata filtering.
- For example, a query for “Java” could refer to the programming language or the Indonesian island. Metadata helps disambiguate.
2.3 Efficient Retrieval
- Searches with metadata filters retrieve a precisely defined set of documents.
- This efficiency ensures that users receive the nearest-neighbour results that match their criteria.
3. Implementation Considerations
3.1 Indexing and Storage
- Efficient indexing of metadata is crucial.
- Systems must handle large-scale metadata efficiently to support real-time retrieval.
3.2 Query Expansion
- Combining metadata filters with query expansion techniques can further enhance retrieval.
- Expanding the query based on relevant metadata terms can yield better results.
Conclusion
Meta-data filtering is a powerful tool for improving document retrieval. By considering additional information associated with documents, we enhance relevance, handle ambiguity, and achieve efficient retrieval. Incorporate meta-data filtering into your retrieval system to provide users with more accurate and context-aware results.
EXAMPLES:
Query: How does Verizon's new service help customers stay in touch at all times?
Without Metadata filtering:
Answer: Verizon's new service, Iobi, acts as a virtual switchboard operator that sends phone calls, voicemails, and emails wherever customers designate. This allows customers to receive and manage their communications effectively, ensuring they can stay in touch at all times.
Response Time:
CPU times: user 134 ms, sys: 6.82 ms, total: 141 ms
Wall time: 2 s
With Metadata filtering:
Answers: Verizon's new service, Iobi, helps customers stay in touch at all times by offering messaging and call-forwarding features that allow customers to receive phone calls, voicemails, and emails wherever they designate. This service merges regular telephone lines with Internet-based features, such as voice messages available through email and automatic call forwarding, providing customers with the benefits of advanced Internet phone systems even if they have only conventional telephone lines and dial-up Net access or no computer at all.
Response Time:
CPU times: user 114 ms, sys: 5.57 ms, total: 120 ms
Wall time: 4.73 s
Query: How does SUN's approach to releasing Java3D differ from its approach to other projects, and what benefits does this new approach offer?
Without Metadata Filtering:
Answers: SUN's approach to releasing Java3D differs from its approach to other projects by involving developers in the evolution of the Java 3D API. They have made the core utilities available under a BSD license and provided the source code for the core Java 3D API, vecmath, core utilities, and program examples on java.net. This new approach offers benefits such as increased transparency, community involvement, and the ability for developers to provide feedback and contribute to the development of Java3D.
Response time:
CPU times: user 138 ms, sys: 7.89 ms, total: 146 ms
Wall time: 3.06 s
With Metadata Filtering:
Answers: SUN's approach to releasing Java3D differs from its approach to other projects in that they have provided the core utilities under a BSD license and made the source code available on java.net. This new approach involves developers in the evolution of the Java 3D API, allowing them to join the Java 3D projects on java.net and download the source code for the core Java 3D API, vecmath, the Java 3D core utilities, and the Java 3D program examples. This approach benefits developers by giving them the opportunity to contribute to the development of Java3D, access the source code, and collaborate with others in the community.
Response time:
CPU times: user 85.4 ms, sys: 3.63 ms, total: 89 ms
Wall time: 4.07 s
Final Conclusions
The field of Retrieval-Augmented Generation (RAG) has witnessed enormous advancements in recent times, enabling more efficient and accurate information retrieval and generation processes. From chunking strategies that break down large documents into manageable chunks to embedding models that can encode semantic information, RAG systems leverage a wide range of techniques to improve their performance.
Indexing techniques, combined with efficient vector stores, play an important role in enabling fast and scalable retrieval of relevant data. Metadata filtering further refines such search processes by leveraging additional contextual information associated with documents, thereby improving relevance and reducing computational overhead.
Reranking techniques, such as cross-attention and fine-tuning, assist in prioritizing the most relevant documents, ensuring that the generation stage operates on the most appropriate information. Multi-query approaches further enhance the capability of RAG systems by decomposing complex queries into multiple sub-queries, enabling more comprehensive and nuanced outputs.
As the field continues to evolve, advanced RAG applications will likely incorporate techniques such as combining multiple retrieval and generation methods, distributed retrieval and generation, and improved evaluation metrics tailored to RAG systems. These advancements will enable more accurate, coherent, and context-aware information retrieval and generation, with applications spanning domains like question-answering, literature search, legal research, and policy analysis.
The future of RAG lies in the seamless integration of retrieval and generation components, leveraging the strengths of both approaches to provide more comprehensive, nuanced, and trustworthy information to users. With continued research and development, RAG systems will play a pivotal role in enhancing our ability to navigate and make sense of the vast information landscapes we encounter daily.